Introduction to artificial intelligence (AI): history and challenges
Artificial intelligence (AI), once confined to the realms of science fiction, is now a transformative technology at the heart of many critical systems. To understand its implications and potential, we need to delve into its history, analyse its technological advances and assess the technical and ethical challenges it poses to our societies.
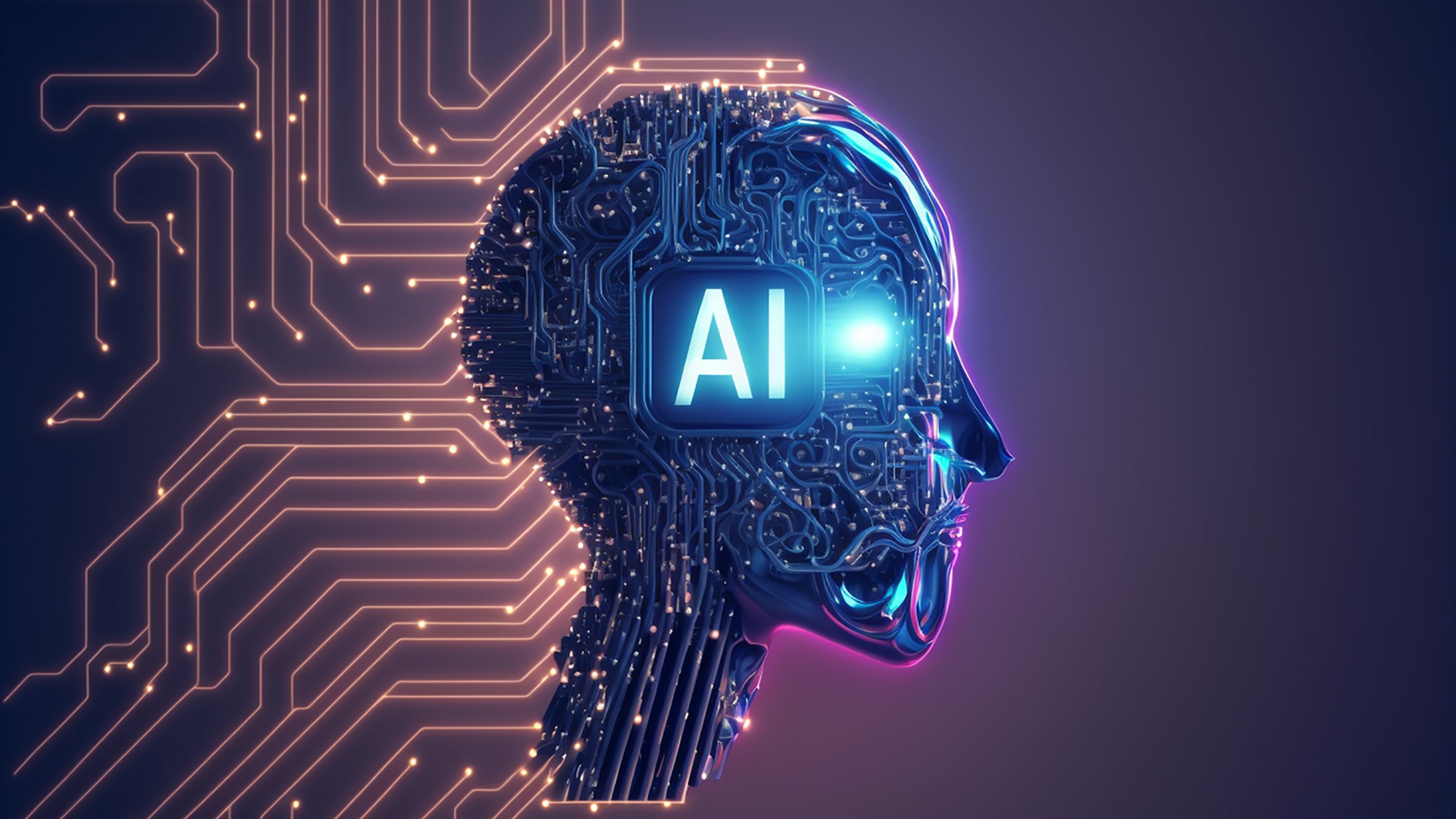
The origins of artificial intelligence: the beginnings of a technical discipline
The theoretical foundations of AI date back to the mid-twentieth century, with work in mathematical logic and cybernetics. But it was in 1956, at the Dartmouth Conference, that the term artificial intelligence was officially adopted. The aim of pioneers such as John McCarthy and Marvin Minsky was clear: to develop machines capable of solving complex problems, learning and even simulating human behaviour.
At the same time, Alan Turing had already set the ball rolling with his famous Turing Test. The idea? To determine whether a machine could behave indistinguishably from a human in a conversation. Although this concept is still debated today, it marked a turning point in the design of so-called "intelligent" systems.
Early research focused on symbolic approaches: algorithms designed to manipulate symbols and solve mathematical or logical problems. Although promising, these methods came up against two major obstacles: the lack of computing power in computers and increasing complexity as tasks became less structured.
Technological transitions: from expert systems to deep learning
Symbolic systems and the first winters of AI
In the 60s and 70s, the symbolic approach (often called classical AI or GOFAI for Good Old-Fashioned Artificial Intelligence) dominated. These systems, based on explicit logical rules, worked well for specific tasks such as playing chess or solving puzzles. But when it came to managing imprecise or unstructured data, their limitations quickly became apparent.
These frustrations led to two AI winters (the 1970s and late 1980s), periods when funding and interest in AI research declined sharply. The ambitious promises made by researchers simply did not materialise as expected.
Machine learning: a new lease of life for AI
The turning point came in the 1990s with the rise of machine learning. Rather than manually coding rules, researchers began to develop algorithms capable of learning from data. The use of statistical techniques such as decision trees and support vector machines (SVMs) has made it possible to solve classification and prediction problems with much greater accuracy.
At the same time, the availability of massive data and the increasing power of computing infrastructures accelerated progress. Algorithms became more efficient as they 'ate' more data.
Deep learning: the revolution of the 2010s
The real revolution came in the early 2010s with deep learning. Based on deep neural network architectures, these models transformed fields such as computer vision and natural language processing. One of the most significant breakthroughs came from AlexNet in 2012, which dominated the ImageNet competition thanks to a convolutional network (CNN) capable of classifying millions of images with unprecedented accuracy.
Advances in models based on transformers (such as BERT or GPT) have since opened up new perspectives in natural language processing. These architectures make it possible to handle complex tasks such as translation, automatic summarisation and text generation, with unprecedented levels of performance.
The contemporary challenges of AI: beyond algorithms
1. Biases in AI systems
AI is only as intelligent as the data it uses. If the training data sets are biased, the models reproduce and amplify these biases. For example, studies have shown that some facial recognition systems perform much less well for groups that are under-represented in the data, such as women or non-white people. These biases can have serious consequences in areas such as recruitment or justice.
Researchers are actively working on solutions: increasing data sets, introducing equity metrics, or adjusting algorithms to detect and correct these biases.
2. Explicability: understanding complex models
Modern AI models, particularly those based on deep learning, are often described as black boxes. Their inner workings are difficult to interpret, even for experts. In critical areas such as health or finance, this opacity poses a problem: it is essential to understand why a decision has been taken. Approaches such as local explicability (LIME, SHAP) or attentional networks help to make these models more transparent.
3. Security and robustness in the face of adversarial attacks
Another major issue is the vulnerability of AI systems to adversarial attacks. Simple disturbances that are imperceptible to the human eye can trick a model into making incorrect decisions. For example, in the case of autonomous vehicles, a minor change to a road sign could cause an accident.
Solutions include mechanisms for hardening models, such as adversarial training, or systems capable of detecting these anomalies in real time.
4. Regulation and technological sovereignty
Although frameworks such as the RGPD have been in place for several years, they are not sufficient to address the technical specificities of AI. The European AI Act currently in preparation seeks to fill this gap, by establishing standards for the use and development of AI. But there are still major challenges ahead, not least ensuring fair competition with American and Asian technology giants.
The future of AI: promises and challenges
Current AI is specialised, but research into general AI is advancing. AGI promises systems capable of solving multiple tasks without specific training, but it raises fundamental questions about human control, safety and ethical implications.
At the same time, advances in foundation models, such as LLMs (Large Language Models), are opening up unprecedented prospects. These multi-task models push back the limits of automation, but require colossal resources and pose ecological challenges.
Conclusion: AI, between maturity and the quest for optimisation
Artificial intelligence has come a long way since its symbolic beginnings. It is now at a critical crossroads: how to maximise its benefits while minimising its risks? For the experts, the key lies in collaborative research, appropriate regulation and the responsible integration of technologies into human systems. AI is not just a tool: it is redefining the paradigms of our society.
Our training courses for Data
Discover our 5 to 10 week data bootcamp to become an expert and launch your career.I
II
III
Registration
Bootcamp
Registration
About you
Introduction to artificial intelligence (AI): history and challenges
remaining space
Next session
from at
Thank you for your application.
remaining space
Next session
from at
Next steps
ApplicationSent to
48h
Telephone interviewAn e-mail will be sent to you
Preparatory exercisePlan a day